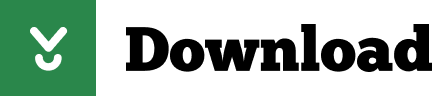
This emulates the cortical decomposition of the HVS using a wavelet transform. The contrast sensitivity and the near-threshold and supra-threshold properties of the HVS were exploited by Chandler and Hemami in their Visual Signal-to-Noise Ratio (VSNR) still image metric. Based on this concept, quality metrics have been developed which emulate the perception process using a two step strategy: near-threshold and supra-threshold. Under these circumstances, it is often more efficient to characterize the artifacts rather than to purely compute distortions. When distortion becomes significant, visual attention is more likely to be attracted by visible artifacts. It has been noted that this improvement is more significant when the distorted content is similar to the original. When HVS properties are exploited, it is generally found that the metric provides enhanced correlation with subjective judgements, compared to conventional distortion measures such as MSE. A similar phenomenon exists with dynamic textures. Masking effects are more evident in spatially textured regions, where the HVS can tolerate greater distortions than in smoother areas. For example, CSF weighting has been employed to reflect the HVS sensitivity across the range of spatial and temporal frequencies, and visual masking-the reduction of perceived distortions at certain luminances and in spatio-temporal textures-has also been exploited. HVS characteristics such as contrast sensitivity and visual masking have been exploited, both in coding and in quality assessment. Bull, in Academic Press Library in Signal Processing, 2014 5.07.5.2.1 HVS-based quality metrics Thus, we model the HVS noise in the wavelet domain as stationary RFs N = where N → i and N → ′ are zero-mean uncorrelated multivariate Gaussian with the same dimensionality as C → i:įan Zhang, David R. We call this lumped HVS distortion visual noise, and model it as a stationary, zero mean, additive white Gaussian noise model in the wavelet domain. As a matter of analytical and computational simplicity, and more important to ease the dependency of the overall algorithm on viewing configuration information, we lump all sources of HVS uncertainty into one additive noise component that serves as a distortion baseline in comparison to which the distortion added by the distortion channel could be evaluated. Although one could model different components of the HVS using psychophysical data, the purpose of the HVS model in the information fidelity setup is to quantify the uncertainty that the HVS adds to the signal that flows through it. In this chapter, we approach the HVS as a “distortion channel” that imposes limits on how much information could flow through it.
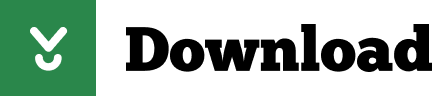